Introduction
With the rapid development of artificial intelligence , Ai has the ability to analyze data and make decisions in a way never possible before. On the other hand, with all of this AI in everyday use, the issue of privacy of information has become a large issue that how the data is collected, kept, and used. The crossroad of AI and data privacy are plagued(harass) with problems, such as hackers, data breaches, and abuse of personal information. And with the development of AI systems, they require and process much more personal data, which in turn can become more risky and unethical. This essay explores the intersection of AI and data privacy, examining the challenges, implications, and potential solutions to safeguard personal information in the age of AI.
Role of AI in Data Privacy
And of course, AI, especially machine learning and deep learning, flourishes on big data. These files may include personal data, including medical records, financial transactions, and social networking activities. All this information is then processed by AI algorithms that can recognize patterns and predict future outcomes and provide tailored services. For example, the AI recommend systems on netflix and amazon use the users data to recommend movies and shows and products that would appeal to each individual.
Although these applications make the experience of the user so much better, they become a great threat to privacy. The AI systems collect and process so much data that it is susceptible to breaches, misuse, and people tampering with it. The second reason is that AI algorithms are so mysterious that one can never know how their personal data is being used or whether or not privacy laws are being followed.
Privacy Risks Associated with AI
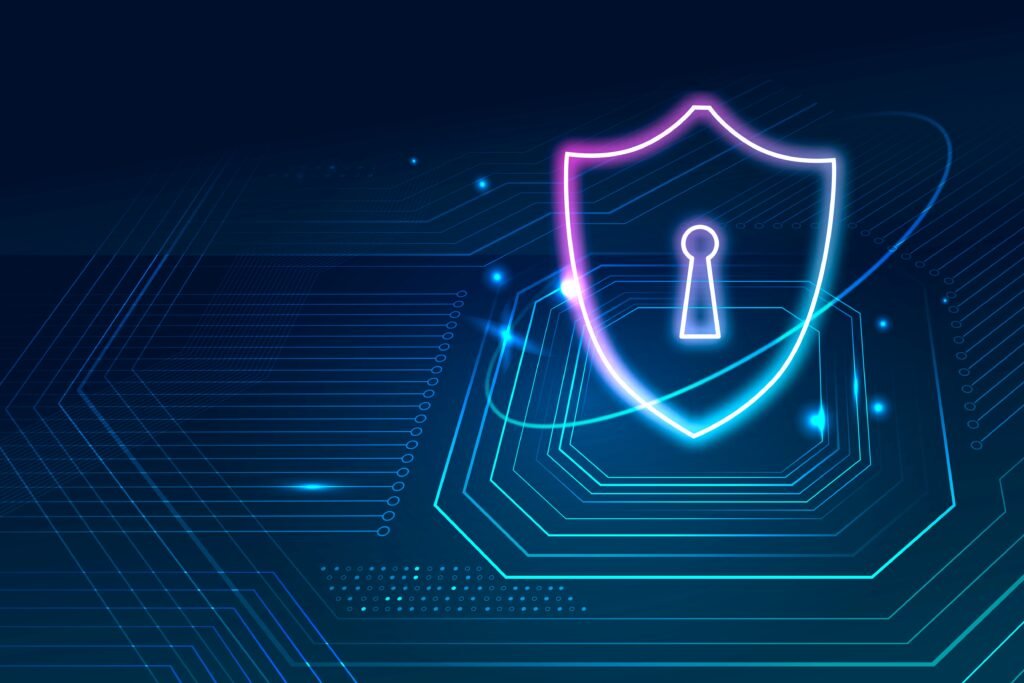
➢Data Exploitation
And it is this ability on the part of AI to collect and process huge amounts of information that sets the stage for data exploitation. That information, once gathered in AI systems, can be used for other things than what the user consented to, like targeted advertising, or even worse, sold to third parties.
➢Identification and Tracking
AI applications, such as facial recognition and location tracking, can identify and monitor individuals without their knowledge. This ability is very scary because of the possibility of surveillance and misuse by both private corporations and government agencies.
➢Bias and Discrimination
However, AI systems can unintentionally reinforce the prejudices found in the training data. As an example, AI-powered job recruitment systems have been shown to be biased against some racial/gender/etc. groups, which leads to discrimination and perpetuates current inequalities.
➢Data Breaches
AI systems are also tempting targets for hackers with the central storage of so much personal information. That leaves data breaches, which could lead to identity theft, financial loss, and loss of reputation.
Regulatory Frameworks and Ethical Considerations
In order to compensate for these privacy hazards, many regulatory structures and moral codes have emerged. The General Data Protection Regulation (GDPR) in the European Union is one of the most comprehensive data protection laws, setting strict requirements for data collection, processing, and storage. It gives people control over their own data, the right to access, correct, and erase it.
Along with laws, morals also play a very important role in keeping information private in AI systems. Organizations need to incorporate the ideas of transparency, accountability, and fairness into their use of AI. Which could be anything from frequent auditing of AI algorithms to strong data security to making sure that AI systems themselves do not reinforce any existing biases or discrimination.
Technological Solutions for Enhancing Data Privacy
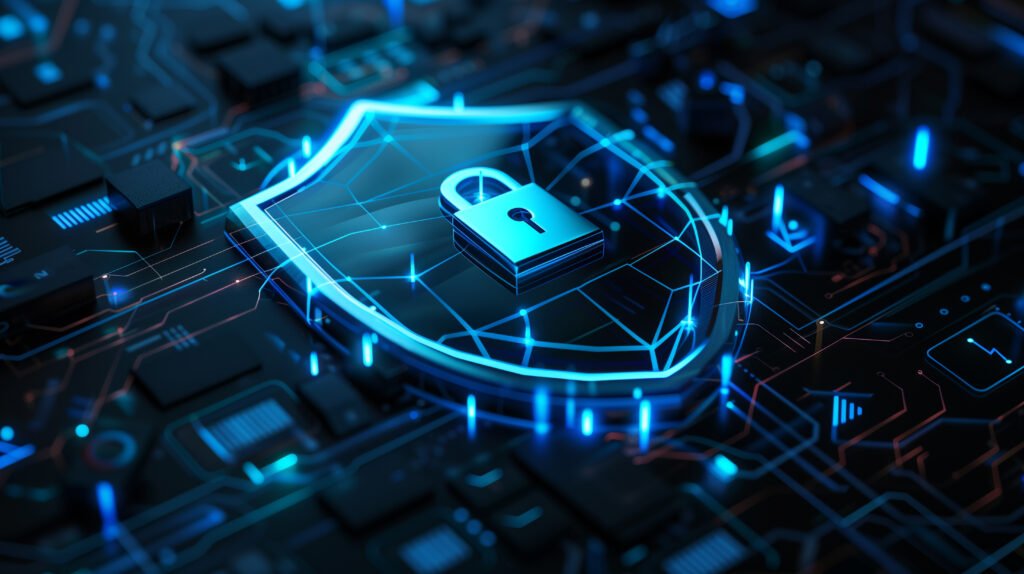
There are many technoligical remedies to the privacy problems that AI can cause, though.
◉Differential Privacy: This method “muddies the water” so to speak, but individual records cannot be identified, yet the data can still be analyzed meaningfully. Apple and Google use differential privacy to ensure user data is kept secure in their AI applications.
◉Federated Learning: Federated learning disseminates the ability to train AI models to the user’s local device, rather than sending raw data back to a central server. This method also minimizes the possibility of data leaks and provides more privacy because personal data is stored on the users machine .
◉Encryption: In this way, encrypting data while it is sitting (at rest) or moving (in transit) guarantees that even if the data is intercepted it is worthless without the key to decrypt it. AI systems must have advanced encryption capabilities to protect any sensitive information that they process.
◉Anonymization: Privacy can be maintained by simply eliminating personally identifiable information from the datasets, and yet the data is still able to be analyzed. On the other hand, it should never be easy to deanonymize that data.
Case Studies
✦Healthcare: AI is going to revolutionize medicine with tailored treatments and early disease recognition. But at the same time, there’s issues with privacy when it comes to AI in healthcare. For example, the sharing of patient data between hospitals and AI companies must comply with regulations like the Health Insurance Portability and Accountability Act (HIPAA) in the United States.
✦Finance: For example, in the financial services industry, there are AI-based credit scoring and fraud detection systems that operate by sifting through huge amounts of personal financial information. Keeping this information private and secure is vital to preserving confidence in the financial system. All the financial institutions should have strong data protection and they all have to abide by the GDPR and the California Consumer Privacy Act (CCPA).
✦Social Media: Social networking sites use ai to study user data and provide customized content. Yet their data collecting practices are so immense that this has caused many concerns for privacy. I’m thinking about the Cambridge Analytica scandal where millions of facebook users personal data was harvested without consent, that shows us how much stricter data privacy regulations need to be and how ethical the use of ai needs to be.
Future Directions
And so will the problems and solutions regarding privacy of information, for that matter. Future research and development should focus on creating AI systems that prioritize privacy by design. This ranges from creating novel secure data processing methods to making AI algorithms more transparent to holding AI systems responsible for what they do.
And so is international cooperation because data privacy is a global issue. With the harmonization of data protection laws among nations, a uniform standard for the protection of personal data in AI can be established.
Summary
AI can have many great benefits to many different fields but it can also be a great threat to privacy. There are many ways to combat these risks, such as strong regulatory schemes, moral issues, and technological fixes. If we make data privacy a priority in the designing and implementation of AI systems we can utilize the strength of AI without infringing on individual rights and losing public faith.